In the rapidly advancing world of cell and gene therapy, viral vectors have become the molecular vehicles driving transformative treatments forward. These engineered viruses—most commonly Adeno-Associated Viruses (AAVs) and Lentiviruses (LVs)—are designed to deliver therapeutic genetic material into human cells, enabling everything from gene replacement and silencing to reprogramming immune cells in the fight against cancer.
Yet for all their therapeutic promise, viral vectors remain one of the most challenging elements of advanced biologics manufacturing. Designing a viral vector is a complex, multidimensional puzzle that requires balancing tissue specificity, immune evasion, gene expression levels, and payload stability, all within the limited capacity of a viral capsid. Until recently, solving that puzzle relied on iterative wet-lab experimentation, random mutagenesis, and large-scale screening campaigns—a process that is both time-consuming and prohibitively expensive.
Today, that paradigm is shifting. The emergence of artificial intelligence (AI) and machine learning (ML) in biotechnology is beginning to revolutionize how we approach viral vector design. Instead of relying purely on brute-force screening, scientists are increasingly turning to computational tools that can predict, model, and even generate novel vector elements in silico—well before they’re tested at the bench. The result is a new era of rational vector engineering, where design cycles are shorter, outcomes are more predictable, and innovation becomes significantly more scalable.
🔬 Traditional Challenges in Viral Vector Engineering
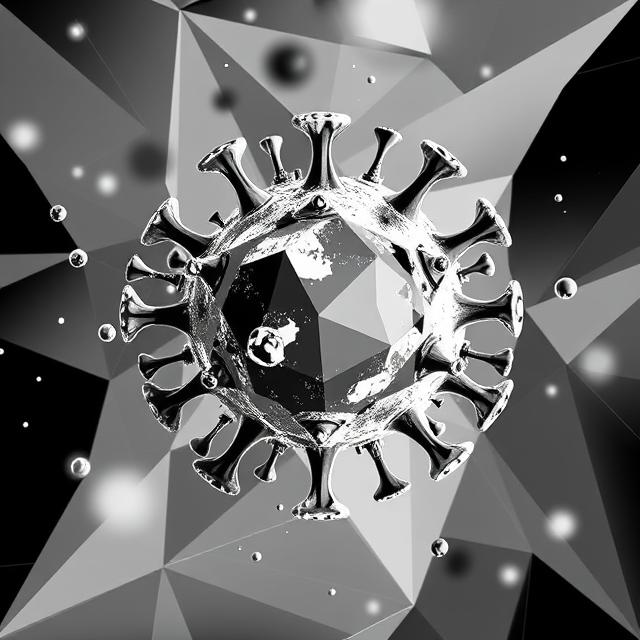
Viral vector development is far from a simple plug-and-play task. At the core of the challenge lies the need to customize the vector’s biological properties to achieve safe and efficient gene delivery. These properties include capsid tropism (the virus’s ability to target specific cell types), immune profile, gene expression levels, and payload constraints—with AAVs, for instance, limited to carrying about 4.7kb of genetic material.
The process has historically involved directed evolution or random mutagenesis, followed by high-throughput screening in vitro and in animal models. Scientists might screen thousands of capsid variants to find a handful that perform well in a particular tissue. Promoters and regulatory elements must also be optimized for strong, tissue-specific gene expression—another trial-and-error process that often takes months or years.
The complexity deepens further when accounting for vector stability, potential for genome integration (in the case of integrating vectors like lentivirus), and the need to avoid immunogenic epitopes that can trigger adverse responses or reduce therapeutic durability. In other words, vector design isn’t just about function—it’s about safety, manufacturability, and regulatory viability.
🤖 AI Enters the Arena: Predictive, Personalized, and Scalable
Enter artificial intelligence. By learning from massive datasets—including previously characterized vector sequences, expression profiles, immunogenicity data, and screening outcomes—AI algorithms can now predict how new vector designs will perform, identify patterns human intuition may miss, and suggest entirely novel variants that are functionally superior to anything created through random trial-and-error.
One of the most impactful areas has been capsid engineering. Companies like Dyno Therapeutics, a Harvard spinout, have pioneered machine learning pipelines that design custom AAV capsids with enhanced tropism, immune evasion, and manufacturability. Dyno’s proprietary “CapsidMap™” platform uses deep learning to explore the vast fitness landscape of AAV variants, enabling the generation of capsids that can more efficiently target specific tissues—like the brain, muscle, or liver—while minimizing recognition by neutralizing antibodies.
Beyond the capsid, AI is also being applied to the non-coding regulatory elements that drive gene expression. Researchers at institutions like MIT and Stanford are using transformer-based neural networks and generative adversarial networks (GANs) to design synthetic promoters and enhancers. These models are trained on vast genomic datasets to predict expression strength and tissue specificity—allowing for the creation of promoters that are compact, tightly regulated, and tailored to the disease context.
Even the payload itself can benefit from AI-based optimization. Algorithms are being used to simulate how a gene construct will fold, predict secondary structure interactions, and flag sequences that might interfere with packaging or expression. This is especially critical for maximizing limited capsid space and ensuring translation efficiency once inside the target cell.
🧬 Industry Leaders & Academic Powerhouses Driving the Shift
The rise of AI in vector design isn’t happening in a vacuum—it’s being propelled by a wave of forward-thinking biotech companies and academic centers building out the tools and infrastructure for the next generation of gene therapy.
Dyno Therapeutics remains a standout, having forged partnerships with giants like Roche, Astellas, and Novartis to design tailored AAV capsids for central nervous system (CNS), ocular, and systemic delivery. Their model-driven approach replaces thousands of lab experiments with targeted AI predictions, drastically reducing time to lead candidate.
AskBio, now part of Bayer, has integrated computational capsid evolution and vector design into its pipeline, combining AI models with high-throughput in vivo validation to push forward both clinical programs and vector IP.
On the academic side, the Wyss Institute at Harvard and the Synthetic Biology Center at MIT are pushing boundaries in synthetic promoter design, using deep learning models to generate modular, compact genetic control systems. These innovations are poised to enhance both viral and non-viral delivery systems across therapeutic areas.
Companies like Krystal Biotech, known for their skin-targeted gene therapies, are exploring AI tools to improve tissue-specific targeting and vector stability, while Orna Therapeutics is blending circular RNA delivery with capsid innovation guided by ML tools.
🔧 Technical Spotlight: Where AI Adds the Most Value
To understand the true impact of AI in this space, it’s worth breaking down where the technology provides the most transformative lift:
- Capsid Design & Tropism Prediction: ML models trained on thousands of AAV capsid variants can predict which combinations of amino acid substitutions enhance targeting to specific tissues, helping design next-gen serotypes without needing full-scale evolution libraries.
- Promoter and Regulatory Sequence Design: AI tools can simulate the impact of sequence changes on gene expression dynamics, leading to the creation of small, powerful, and tissue-specific promoters—critical for space-limited vectors like AAV.
- Payload Stability and Folding: AI models can forecast whether a gene will fold correctly when expressed, identify problem motifs (e.g., hairpins, repeats), and suggest codon-optimized versions for expression in specific hosts.
- Immunogenicity Prediction: Deep learning algorithms can screen for potential B- and T-cell epitopes in capsid proteins and recommend substitutions to reduce immune recognition while preserving function.
These AI applications are enabling a shift from random to rational, from empirical to predictive, and from slow to scalable.
🔮 Looking Ahead: Toward Fully Computational Vector Design
As AI continues to mature and integrate with wet-lab platforms, we’re approaching the reality of fully in silico viral vector design pipelines. In this future, researchers and CDMOs will be able to simulate how a vector will behave in a patient before it’s ever produced, optimizing every component—from capsid to promoter to payload—in advance.
This will enable:
- Faster development cycles, accelerating time-to-clinic by months or even years.
- More personalized gene therapies, tailored to a patient’s tissue type or immune profile.
- Better targeting and expression, enhancing efficacy while minimizing off-target risks.
- Reduced cost and attrition, particularly in early-stage screening and preclinical validation.
💡 Final Thoughts
The intersection of artificial intelligence and viral vector engineering is one of the most exciting frontiers in biotechnology. We’re moving beyond the limits of biology as a black box—and toward a future where vector design becomes a matter of computation, simulation, and intelligent iteration.
AI is not replacing human intuition or creativity—it’s enhancing it. It allows scientists to explore more possibilities, ask better questions, and build smarter systems. For gene therapy, this means safer, more effective, and more scalable treatments. For CDMOs and developers, it means new levels of agility and precision in one of the most high-stakes fields in medicine.
The viruses we once feared are now the vehicles for curing disease. And with the power of AI, we’re learning to write their code better than ever before.